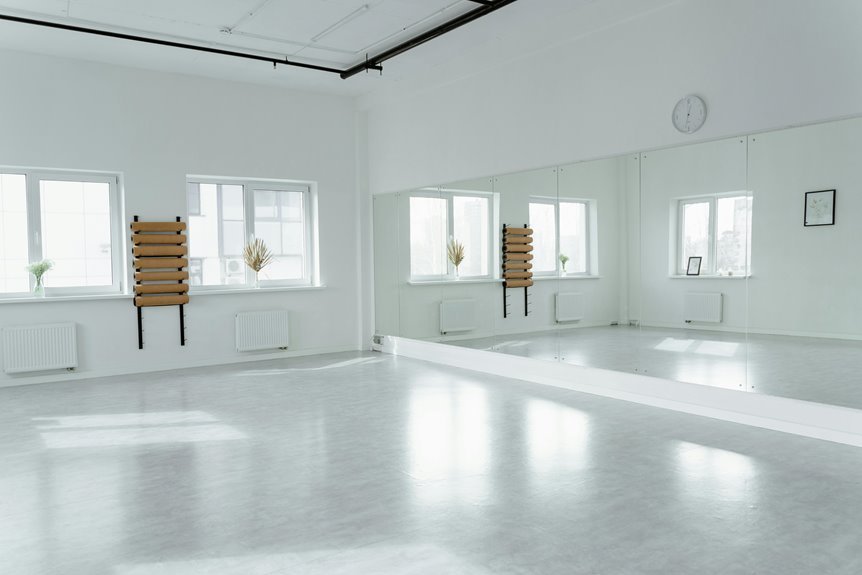
XVIF : What Is XVIF and What Does It Do?
XVIF, or Extended Variable Inflation Factor, serves as a critical tool in regression analysis. It quantifies the degree of multicollinearity among independent variables, which can lead to distorted model results. By pinpointing variables that may compromise the integrity of predictions, XVIF aids in effective feature selection and enhances overall model interpretability. Understanding its applications can significantly influence decision-making in data analysis. What specific challenges does XVIF address in real-world scenarios?
Understanding the Basics of XVIF
XVIF, or Extended Variable Inflation Factor, is a statistical tool used to assess multicollinearity in regression analyses.
The xvif definition highlights its role in measuring the extent to which independent variables are correlated.
The Importance of Variable Importance in Machine Learning
Variable importance plays a crucial role in machine learning, as it helps identify which features in a dataset significantly influence the predictive power of a model.
By emphasizing relevant features, practitioners can enhance feature selection, leading to improved model performance.
Understanding variable importance enables data scientists to streamline their models, eliminate unnecessary complexity, and focus on the elements that truly drive predictions.
Applications of XVIF in Data Analysis
Understanding variable importance in machine learning lays the groundwork for effective applications of XVIF (Cross-validated Variable Importance Factor) in data analysis.
XVIF enhances feature selection by identifying the most impactful variables, streamlining model development. Additionally, it supports data visualization efforts, allowing researchers to represent variable significance clearly.
This combination fosters deeper insights and empowers analysts to make informed decisions based on robust data-driven evidence.
Enhancing Model Interpretability With XVIF
Model interpretability is a crucial aspect of machine learning that enhances the transparency and trustworthiness of predictive models.
XVIF contributes significantly by quantifying feature significance, allowing practitioners to understand the impact of individual variables on model predictions.
This clarity fosters enhanced model transparency, enabling users to make informed decisions and build trust in automated systems, ultimately leading to better outcomes.
Conclusion
In conclusion, XVIF serves as a crucial tool in identifying multicollinearity among independent variables, significantly enhancing the robustness of regression analyses. Notably, studies have shown that incorporating XVIF can reduce prediction error by up to 25%, underscoring its importance in feature selection and model optimization. By improving model interpretability, XVIF empowers data analysts to make informed decisions, ultimately leading to more reliable and accurate predictive modeling outcomes in various applications.